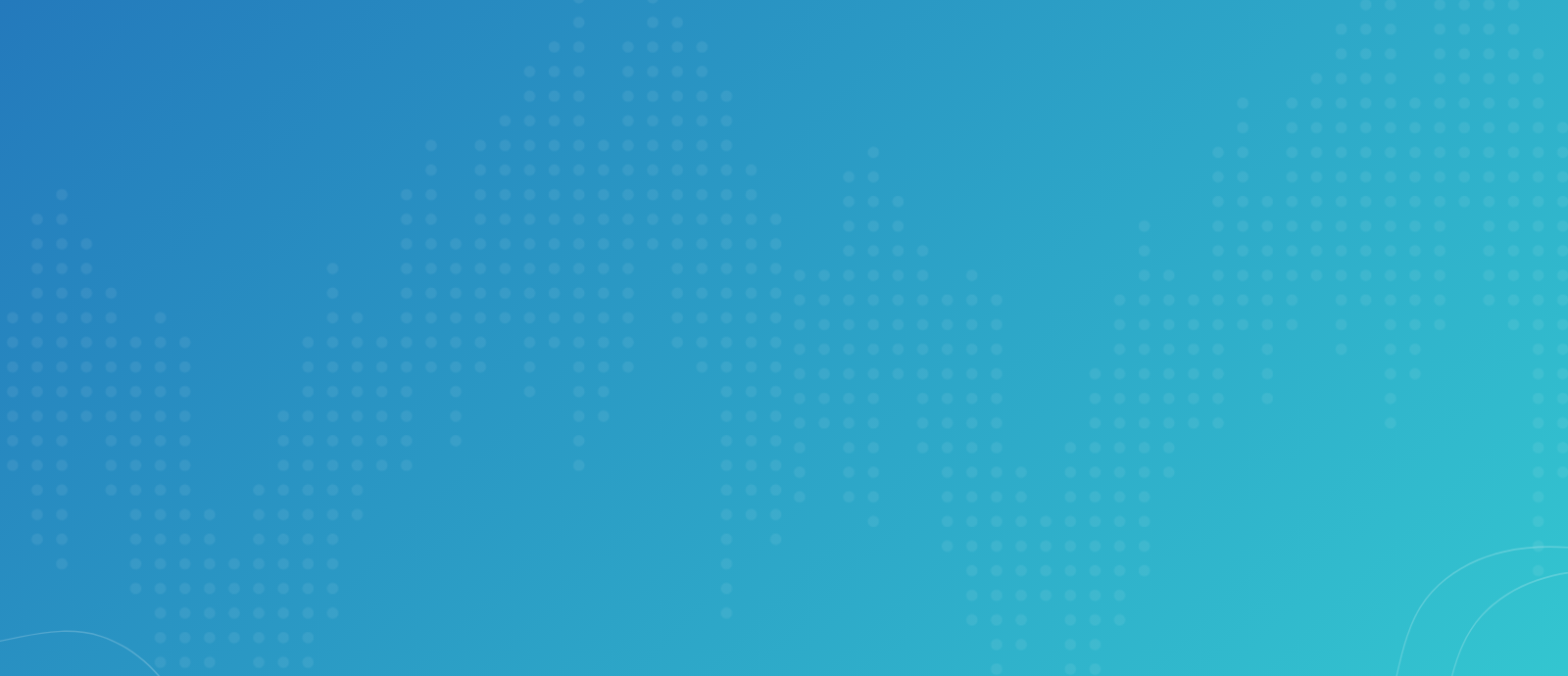
Webinar: How State Contexts Impact Population Health
Date
December 2, 2024
In this Nov. 14, 2024 webinar, two distinguished researchers discussed how U.S. state policies and systems can affect racial and regional inequities in health and longevity.
- Tyson H. Brown (Duke University) focused on innovative and best practices for measuring and modeling state-level structural racism to advance aging health research.
- Jennifer Karas Montez (Syracuse University) summarized findings from recent studies that have connected the dots between changes in states’ policy contexts in recent decades and changes in population health.
This webinar was hosted by PRB and the Coordinating Center for the Centers on the Demography and Economics of Aging and Alzheimer’s Disease and Related Dementias, with funding from the National Institute on Aging.
Transcript
Mark Mather, PRB: Hi everyone, thank you for joining today’s webinar on how state contexts impact Population Health. I am Mark Mather. I’m with the Population Reference Bureau, or PRB, and I work in collaboration with the Coordinating Center for the Demography and Economics of Aging and Alzheimer’s Disease and Related Dementias at the University of Michigan. And our goal is to help publicize research on health and well-being, especially among older adults. I also want to acknowledge the National Institute on Aging for making this webinar possible, and my PRB colleague, Toshiko Kaneda, for helping us to organize this event.
I’m excited to introduce our two speakers today. We have Dr. Tyson Brown, who’s a Professor of Sociology, Associate Professor in medicine, and Associate Scientific Director of social sciences of the Duke Aging Center at Duke University and Dr. Jennifer Montez, who is a Professor of Sociology, Gerald B Kramer faculty scholar in aging studies, Director of the center for Aging and Policy Studies, and Co-director of the Policy, Place and Population Health Lab at Syracuse University.
We’re also going to put two links in the chat, I’m not going to read their long bios, but if you’re interested in learning more about the speakers and their research, we will be putting links in the chat where you can get more information. And finally, just a couple of housekeeping notes; we’re going to hold the Q&A until the end. But if you do have a question, you can type it into the Q&A box at any time during the webinar. And finally, this webinar is being recorded, we will send you a link to that recording after the event, probably within a day or two. And with that, I will go ahead and turn it over to you, Tyson.
Dr. Tyson Brown, Duke University: Well, thank you so much. I look forward to hearing your feedback during our presentation. I will go ahead and just start sharing my screen. Again, thank you so much for inviting me to present my work, and I’m really excited to talk with you all today about how we can operationalize state level structural racism and its impact on population, health and aging. And as a race scholar, gerontologist, and population health scientist, you know, the primary focus of my research is on quantifying and mapping structural racism, as well as estimating its impact on aging and health equity. And so today, I’ll be giving an overview discussing what I see as important theoretical and methodological issues, as well as promising avenues to address them, and in doing so, advance the scientific study of structural racism and health.
So, before discussing the role of states, I think it’s really useful to zoom out to highlight how various frameworks can help us better understand how social context influence health. So, the social ecological model emphasizes that population health is shaped by complex interplay of social factors across multiple levels, from individual behaviors to broader societal forces. And this model highlights how health outcomes are not solely a product of individual choices, but are influenced by interpersonal relationships, community structures, institutional policies, and broader sociopolitical contexts. And by analyzing each of these, we gain insights into how policies, societal norms, and community structures can either benefit or harm population health. And this is true for many social forces, including structural racism.
And so, most of the research on links between structural racism and health have focused on structural racism at the neighborhood or the county level, which are really important context and really sort of the micro-level, or rather the more proximate contextual sources or determinants of health. However, other special units and policies such as states have received comparatively less attention. And this is a really major limitation in literature, because U.S. states obviously are important legal, administrative and political units and states have always provided an important context for racial stratification, including the roles that they most obviously played with respect to chattal slavery, Jim Crow and anti-miscegenation laws. So importantly, states continue to have a great deal of autonomy, and they’re heterogeneous in terms of the racialized policies and practices. This is evident with respect to things like disenfranchisement laws, aggressive policing practices, punitive sentencing, as well as the expansion or contraction of safety net resources.
And so, as a result, my research focuses on conceptualizing states as racialized institutional actors that influence population health.
So, in several recent studies, my research team scopes the literature on social racism to really identify the central tenets of structural racism theories and draw upon them to study state level structural racism. We highlight how the analytical crux for measuring structural racism, which is a complex, interconnected and dynamic network, really lies in examining its features and mechanics that undergird racial inequalities.
And so, failure to align measurement tools with these core features compromises the validity of research. Because measures that are incongruent with salient aspects of structural racism can distort findings. So, to address this issue, we draw on interdisciplinary theories and evidence to really dissect core features of social racism, with a focus on their implications for measurement and modeling.
And so, our studies on and the broader literature really underscore the importance of measuring modeling core features of structural racism, including things like the fact that it’s a distributional system, involves relational power dynamics, manifestations of racial inequities, that it’s a multi-level phenomenon operating at macro and micro level contexts, that it’s multifaceted and interconnected in nature. And this plays out across societal domains, that there are specific racial actors implicated, that it’s an institutionalized phenomenon that it involves racial schemas, logics, as well as socio historical context and intersections with other systems of oppression. And so structural racism is embedded in major political, economic, medical, criminal, legal and numerous other social institutions and aspects of society. So, in these forthcoming articles that are listed here, we offer concrete recommendations for conceptualizing, measuring, and modeling structural racism in ways aligned with theory. And so, the aims of these studies is to provide field guides for rigorous, theory driven measurement approaches, proposing best practices for the scientific study of structural racism in health research.
And to provide a couple concrete examples of ways to measure structural racism, as well as its effects on health, I’ll briefly describe several recent studies in this vein. And so, the first one addresses two main research questions. The first is, does state level structural racism across societal remains reflect an underlying latent construct? And latent constructs are phenomena that are not directly observable but can be estimated through statistical approaches that really capture manifestations of the phenomena. And then secondly, the question is structural racism associated with worse health outcomes among Blacks and whites in the U.S.
So, to examine variability in state level structural racism and its relationship with health among Black and white adults, we combined indicators of structural racism from several sources of publicly available data. And then we link these to geo coded individual health and demographic data from the Health and Retirement Study, as well as CDC data on COVID mortality, as well as the Behavioral Risk Factor Surveillance study. And so, this table shows each of the domains and indicators of structural racism that we examine. We use publicly available data to examine structural racism for the year circa 2010, spanning several domains including the criminal legal system, education, economic resources, political participation, as well as residential segregation.
And so, I should mention that this measurement approach is informed by theoretical perspectives as well as previously validated measures of state level structural racism.
So, the next step is to test whether state level structural racism reflects this underlying latent construct. And so, we’re especially interested in measuring structural racism in ways that are in line with structural theories, as I mentioned. And a latent variable approach is well suited for minimizing measurement error and capturing conceptual properties of a complex system that’s difficult to quantify or directly measure, such as structural racism. And so along those lines, the nine indicators of structural racism that I mentioned were used to develop a latent measure of structural racism. And we used confirmatory factor analysis to measure the extent to which structural racism across these domains are reflective of an underlying construct of structural racism and doing so we systematically evaluated model fit using varying model specifications related to correlated errors and also dimensionality. This figure here illustrates the factor structure of the latent construct. These correlated errors are all intended to address common sources of variation that are independent of the effects of structural racism on the various indicators. And so all consider the measurement model that allows for other common sources of variance in the indicators that are largely conceptually motivated, has a good fit with the data, and it generates reasonable parameter estimates. Furthermore, this measurement approach is largely consistent with many of the theoretical tenets that I outlined above. And I should note that we made this measure publicly available from the journals website in case you’re interested in exploring. So now we’ll turn to addressing the second research question, which is how does structural racism shape health among Black and white adults? Eco social theory has become a leading framework for understanding how macro level discriminatory environments impact health, and the theory suggests that structural racism has deleterious effects on Black people’s health. That part’s pretty much straight forward. We would anticipate that from the theory. And there are competing hypotheses about how structural racism may influence the health of whites, with some suggesting that whites benefit from sexual racism, while others are positing that they’re harmed by it, and others suggesting that their health may be unaffected by structural racism. I’m glad to talk more about these competing hypotheses during in more detail during the Q&A, if you’d like.
But let’s go ahead and jump into the results. What’s going on here? I’ll explain it in just a second. But one of the things I want to mention is that, you know, since replication is a hallmark of good science, we examine the relationship between structural racism and six different health outcomes in both the Health and Retirement Study and the Behavioral Risk Factor Surveillance study. For a little bit of context, the HRS is considered one of the premier data sources for studying health among adults over the age of 50 in the U.S. is nationally representative over samples of Black adults and the birth. This is the largest health survey in the in the United States. So, you have over 300 respondents. So, you’ve got tremendous statistical power. And so, we found remarkably consistent results across health outcomes and across the two data sets. And six out of the six cases, you can see that there are that higher levels of structural racism are predictive of worse health among Black people, remarkably consistent. But we see a very different story for whites. And one instance, structural racism exposure at the state level is predictive of better health for whites and in five out of six cases, there is no statistically significant relationship between structural racism and whites’ health. So, you find, you know, shockingly consistent results and just, you know, these health outcomes are some of the most commonly used, especially when studying older adults. And so, I think that there’s this, you know, there’s a real signal that we’re picking up. And we had much more confidence in our findings because it was so consistent across these health outcomes and across these health studies. So, in a recent study, we also examined the relationship between a latent measure of structural racism and Black-white inequities in Covid 19 mortality rates. Right.
So, the regression estimates indicate that the relationship between social racism and Black, white inequality in Covid 19 mortality is positive and statistically significant, both in the bivariate model and net of covariates. And so, this again is a state level measure and collectively the findings you know, I would argue that the collectively our findings across these studies suggest that our latent variable approach has really strong predictive validity and we actually did measure it against benchmarked it against other metrics such as sort of a summative index that assumed equal weighting of the indicators and did not take into account correlations between their errors when we found that the latent measure actually explained more variation in health outcomes and also explained significantly more variation of any of the individual level indicators. And so that again, provides some evidence that there’s really there are some real benefits to taking a latent measurement approach. So, I think it’s also essential to measure and map cultural forms of racism that reflect racial schemas, logics and practices.
So broadly you know, we really need to be considering the roles of things like racialized violence, animus, resentment, hate speech, and biases, all of which have been shown to vary by place including across states. And so, in addition to well-established survey data resources, we should also be utilizing data and methods to capture utilizing, you know, more innovative data measures to capture anti-Blackness through geo coded data from things like internet search engines and computational approaches, scraping websites and even experiments. So, this map shows the spatial distribution of a latent measure that Reid DeAngelis and I are developing using these types of data and measures. And preliminary results show that it’s predictive of population health inequities. And so, we’re really encouraged about the possibility of combining these latent measures of racial schemas and logics, sort of cultural measures with the more institutional measures and looking at how the interplay between them and their relative contributions to population health inequities.
I should also mention that in a recent study published in the Journal of Health and Social Behavior, Patricia Home and Brittany King and I, we introduced a state level structural intersectionality approach to population health. And what it does is it really demonstrates an application of social intersectionality using administrative data sources similar to the ones that I discussed in the previous studies. To examine the relationship between macro level structural racism as well as structural sexism and economic inequality and looking at how they interplay between them, as well as their joint and individual contributions to health inequities across U.S. states. Right. And so, this study, I think, can really serve as a springboard and a data source for similar studies that are aiming to extend this research, in studying how state level structural, intersectional oppressions differentially shape health outcomes for various demographic groups. I should also note that numerous studies also really highlight the dynamic role that policies and politics play in shaping health inequities.
Obviously, Jennifer Montez is a new data set, is an excellent resource for studying the role of states in this regard. There are also some studies by biomedical engineer Jackie John and other colleagues that used novel, a novel database on racism related laws which has been shown to predict health. So, these and other data sources on racial policies such as three strikes laws, welfare reform, banning critical race theory and racialized disenfranchisement can really serve as important resources for research on health inequities. And, you know, the history of structural racism in the U.S. has really important implications for how we should really be approaching the measurement of it and quantifying its effects on these inequities. And there is suggest that historical racism directs, constructs and continues to mold contemporary structural racism as well as health outcomes. And so, in that vein you know, there have been several empirical studies that have shown that polities that had larger enslaved populations in 1860, have greater present-day inequities and poverty and economic mobility and also higher levels of contemporary pro-white bias, and that these can be linked to contemporary health outcomes as well, and that historical redlining practices underlie contemporary residential segregation patterns. This is becoming a well-documented social fact, as well as the fact that New Deal policies expanded the white middle class and are directly implicated in modern Black-white inequities and wealth. And so, it really shows the long arm of history in shaping contemporary health outcomes and moving forward. I think a couple of examples of measures to consider include variation and exposure to things like slavery, Jim Crow, lynchings, anti-miscegenation laws, stunned downtowns, the number of folks who are doing really good work in that space. We can think about exclusion from the economic benefits of the New Deal and GI bills, as well as racialized voter suppression.
And so, in many respects, I think what this literature is showing is that what’s past is prologue. And we’re seeing this with new laws, for example, the disproportionately disenfranchised Black and brown Americans. And in closing, I’d just like to highlight a couple, what I see as exciting opportunities for future research that I think will really advance our understanding of the links between racism and aging, health inequities, which I think can be used to inform ultimately efficacious racial equity solutions. So, for example, future studies should really better utilize longitudinal data to better understand the temporal dimensions of the relationships between structural racism and health such as the potential impacts of things like sensitive periods, durations of exposure, as well as causal effects. Right.
And moving forward, you know, I plan to use system dynamics modeling to explicitly model interdependence between forms of structural racism as well as feedback loops and lagged effects. I think that’s a really exciting area that we can start to really overlay temporal dimensions and a life force perspective with our understanding of the processes that ultimately lead to these wide health inequities that get larger and larger throughout the life course.
And also, multilevel research are really needed in this space in order to better understand the cross level structural races and linkages and the joint effects on racial stratification. So obviously, my talk here has been highlighting the role and really funny evidence of the role of states, which are really key. I hope that made that is clear, but of course, other levels also matter, right? and ultimately, you know, this is multifactorial and we should really be thinking about how structural racism across different levels, including state level influence, health.
And then, you know, as the data ecosystem is rapidly expanding, scholars are increasingly calling for a wider use of new sort of big data sources and social science research. And so, I’d just like to echo and expand upon these calls by recommending the use of these new approaches to address salient new research questions about how structural oppression shapes racial inequality.
And so harnessing digital trace data is especially useful as traditional data sources become increasingly costly, increasingly logistically complex and are becoming less representative of the target populations due to declining response rates as well as other selective forces. Also, moving forward, you know, I’d recommend testing the extent to which structural oppression affects inequality indirectly through intermediary social pathways such as unequal access to resources, things like education, income, health care, autonomy, and also exposure to risks, things like toxins, housing instability, victimization, as well as involvement in the criminal legal system and other pathogenic social conditions.
And so ultimately, this is really important. And to do this, we really need to address the and build upon really strengthen the data infrastructure on linking structural racism to health and the current data landscape for structural racism is rather confusing and scattershot.
As I alluded to at the beginning of the talk, you know, we’re willing to build a publicly available data infrastructure on structural racism to catalyze future research on its effects. And creating a data hub will reduce inefficiencies by increasing data sharing, coordination and innovation and so it’s essential that the data include contextual information at multiple spatial and temporal scales, obviously, including the state level. And I see a number of challenges and opportunities to really build a user-friendly data resource like this.
And currently, the process of linking existing data sets to contextual data can be cumbersome, but there are some wonderful opportunities and some recent examples of innovations that reduce these barriers and make it a lot easier, which Professor Montez will be discussing with us. So, I look forward to the Q&A.
Mark Mather, PRB: Great. Thanks so much, Tyson and as a reminder, if you do have a question, we have a Q&A box. So, I encourage you to type your questions into that box at any time. We’re going to take all of those questions at the end of the webinar. So, I will now turn it over to you, Jennifer.
Jennifer Karas Montez, Syracuse University: Great. Thank you. Tyson, I really appreciate your presentation. I love the historical perspective. It’s so important. And I can’t wait to read the 2025 annual review piece.
So, what I’m going to do with my time is give a high-level summary of some of the work that’s been going on using U.S. states to understand why U.S. life expectancy has not been keeping pace with other high-income countries over the last several decades. I don’t know if you can see this thing here where I’m going to try to hide it. Okay, great.
So, this work I’ve been involved in for several years now with this wonderful team. And I want to acknowledge also the funding that we received from NIA to do this work. So, I want to start off by just talking about why we’re focusing on states and state policies. If our goal is to understand what’s going on for the U.S. overall. Um, Just touch on some of the key learnings that we’ve uncovered over the last 5 or 6 years. Talk about how did we get here? Like, how did state policies end up being so important in explaining U.S. population health? And then where do we go from here? So, why are we focusing on states to understand U.S. Life expectancy. Well, this figure you’re looking at here has a line, a wiggly line for all 50 states. It shows their life expectancy trends from 1959 to 2019. And I pointed out Connecticut and Oklahoma, and I’ll do that a lot during the presentation because there are really interesting case study pretty illustrative of what’s happening. So, you can see these two states that we think of today is wildly different in so many ways, they weren’t always that different. So in1959, these two states had the exact same life expectancy. But you can see they’ve taken very different trajectories over this time period. And you’ll notice that, you know, there are some states, like Connecticut who, you know, are approaching, you know, 80, 81, 82 in terms of life expectancy. But there’s a lot of states that have kind of flatlined, if you will, since the mid-80s. And our thinking, our team’s thinking is that if we can explain why we have this divergence in life expectancy across states and why we have so many states that just really haven’t made progress in decades, then that gives us another piece to the puzzle in terms of why U.S. life expectancy is not doing what we’d like it to do.
So, I want to give you one other view of the same data, and in this view, instead of plotting all 50 states, I’m just going to plot the range in life expectancy across the states for every year. And this is what it looks like. And this data runs from 1970 to 2014. Because the study that I’m using this from examine those years. But what I want you to see here is that until 1984, states were actually becoming more alike in terms of their life expectancy. The range was shrinking. And in 1984, something happens and the range and life expectancy just keeps getting bigger and bigger and bigger. And if I were to continue this chart out through present day, you would see that range just grow year after year after year. So, you know, there are a number of explanations, a number of hypotheses for what might be happening here.
Our team has been focusing on the possibility that this divergence in life expectancy across states might be partly explained by the divergence in policies across states. So let me give you a couple of visuals of how states have diverged in their policies. So, this chart, it’s a little messy at first. It has 50 arrows. There’s an arrow here for every state. It’s not very important to figure out which state is which arrow. What these arrows indicate is how each state overall policy context has changed between 1970 and 2014. So, Jake Greenbank is a wonderful political scientist, created this overall measure of state’s policy context. And so, what I’m showing you here is where each state started on that measure in 1970. That’s the beginning of every arrow. And then where each state ended up in 2014 on that measure. And that’s the arrowhead. And so, what I want you to see is that we’ve got most states moving away from the center in terms of their overall policy context. Okay. So, they’re diverging just like states life expectancies are diverging. And again, I picked out Connecticut and Oklahoma so that you can see, you know, in the 70s, these states weren’t that different in terms of their policy context, but they sure are today. So, you might be thinking, well, give me some examples of how are these policies changed over time. So, I’m going to give you just four examples. This summary measure you’re looking at here has over 120 policies all wrapped in it. I’m just going to pull out four of those, four that we know are important for population health. So, let’s take minimum wage and earned income tax credit. Again, two policies that have pretty good evidence that these are important for population health. You can see in 1990, there’s effectively no difference between these two states. In in 1999, neither state offered an ITC. So, there’s absolutely no difference between these two states in these two very important economic policies. But by 2019, you can see these two states are wildly different in these two core economic policies. Let me give you one more example. I’m going to show you how these states have differed in terms of two very important health behavior policies. So, in 1990, these two states had almost identical taxes on a pack of cigarettes and pretty similar number of laws meant to increase the safety of firearms. But by 2019, these states, again wildly different. And it’s not just that a state like Connecticut in blue is moving in one direction in states like Oklahoma or just stuck. But you can see as states like Oklahoma are actually rolling back some of these policies that we know are good for population health. And you can see this most clearly in the firearm safety laws here, where the number of those laws has been rolled back in Oklahoma. So, let’s go back to this chart then. Now what I want to do is give you one more view of how state policy context have changed during this time period. So, what I’m going to do is I’m going to again take this overall summary measure of states policy context, and I’m going to plot the range across the states for every year in that measure. And this is what it looks like. So again, in 1970 until 1981, states were becoming more alike in terms of their overall policy contexts. I mean, it’s almost unimaginable today. But then after 1981, they become increasingly different, increasingly polarized, increasingly divergent. Whatever term you want to use in terms of their overall policy context. And so, if you were here five minutes ago, you’re thinking, I have seen a pattern similar to this before, and you have in fact. So, what I have here is now overlaying the two charts that show how the range in states policy has changed over time.
And the range and states life expectancy has changed over time. So bottom line, as states policy contexts are becoming more similar so were states. Life expectancies three years after states becoming start becoming more dissimilar in their policy environment, states start becoming more dissimilar in their life expectancy. So, at this point in our team’s journey, we thought we had developed some pretty compelling descriptive evidence that there’s a relationship here now. Is it causal? That’s what we wanted to find out. So, I’m going to walk you through some pretty high-level summaries of what we have. Think we’ve learned so far about whether that relationship is more than just a correlation.
So, we’ve found that actually U.S. life expectancy trends would have been significantly steeper if state policies hadn’t changed the way that they did. And that if we were to change all state policy, so all 50 states, if we were to change them all, to have either a very liberal or a very conservative policy context, we could alter us life expectancy by about 2 to 3 years, which is huge. That would put the U.S. about average among its peer countries, as opposed to being very securely at the bottom right now.
Changing state policies in this way would also alter the number of working age deaths each year by about 220,000, again, a very substantial number. So, in more recent work, we’ve been trying to put a more a finer understanding of where are these effects taking place for which causes of death are these effects happening and can we better understand the time lags under which these policies might have an effect? Do we see that policies have an immediate effect on working age mortality? Do we see that it takes a year or two years? How many years does it take? And so, I’m just going to summarize a piece of those findings. So, what you’re looking at here is how much the mortality rate of working age women would change if a state policy changed. And this is specifically CVD mortality among working age women. So, a lot of dots here. So let me walk you through what’s going on. So, look at the far left under the criminal justice policies. So, our analysis suggests that if we were to examine what would happen to working age women, CVD mortality during the very same year that a state’s criminal justice policies went from very conservative to very liberal. That was a lot of words. Let me say it again. So again, this is what we estimate.
What happened to working age women’s CVD mortality, If a state changed its criminal justice policies from conservative to liberal. So, we find that if we were to examine that in the very same year that that that policy was made, we would get a non-significant change in women’s CVD mortality. A year later, we start to see an effect, and within three years later, we start to see a significant reduction in women’s CVD mortality.
So, what this is saying is that if you believe these counterfactual analyses that it takes a while to see the benefits, the mortality benefits of moving from a conservative to a liberal criminal justice policy environment in the States. I’ll just walk you through a couple of other policies, gun safety policy. We find that we see almost an immediate effect in the reduction of women, CVD mortality when gun policies go from conservative to liberal.
And that effect seems to be stronger with each passing year. But there are other policies like health and welfare where we see an immediate effect, but over time that effect attenuates. So, what we take away from an analysis like this is that. The timing matters in our studies. And we don’t often do a very good job of taking timing into account. But imagine the implications. If I were to have only done this study examining no lag or a one-year lag, I would have walked away saying some policies don’t matter when they actually do or saying some do when they actually don’t matter after a given period of time.
And the other thing to take away from this is that not only do we need to pay more attention to it these lag times, but the appropriate lag time might depend on the specific policy that some policies just may take a longer time period to see a population health effect than others.
Another high level finding from our work is that the policies that have changed and polarized the most since the 80s happened to be the same policies that have the strongest associations with life expectancy and working age mortality, and those policies are mainly around labor and firearms.
And then the last finding I want to highlight, and this is not from our group, but it’s important. And I and I’m anticipating some of your questions later on, is that the growing disparities in working age mortality across states do not appear to be due to the changing socio-economic composition of a state’s populations.
So that’s, again, a very high level, a very selective set of what we’ve learned so far. So, what I want to do now is talk about how did we get here. And I want to do this because I want to make sure you, you leave this session realizing that the changes in these state’s policies, these are not exogenous changes, right? There are forces behind the scenes turning the knobs. So, if we want to move our analysis, you know, up the causal chain we need to be looking at who’s changing the knobs and why are they changing them. So, I’m going to point out, maybe my thing. It’s not moving. Okay.
So, I’m going to point out four interlocking forces. And I’ve got several citations down here. Really great in-depth books that you can read on this by sociologists, political scientists and others, a historian. And if you want just the quick and dirty that the very last article in the Milbank Quarterly covers these, these four issues.
So how did we get here? Four things really come together. One is the devolution of federal authority to the states, where states have gained increasing policymaking authority, especially since the Reagan administration. The other thing that has been happening that has given states this increasing power is there has been a proliferation of states enacting preemption laws to take away local authority, to do any number of things from raise the minimum wage, to mandate paid leave, to banning fracking. And so not only have states been given this additional authority by the federal government, they’ve been taking away authority from the states at the same time. Now, those two trends are not in and of themselves problematic but as a result of that, states have made very different decisions about their policies’ environments.
Some of those decisions have been unduly influenced by corporations, their interest groups and wealthy donors and some of the influences coming from the nationalization of political parties. And what I mean by that is, you know, we used to have a Republican version of Oklahoma and a different Republican version of Texas. But now there’s increasingly a Republican version across the U.S., just like there is an increasing Democrat version of policy context across the U.S. And I’m just highlighting this very quickly and again, I’ve given you some citations if you’re interested in digging into that further.
So, I want to conclude with talking about where do we go from here? And some of these recommendations are going to echo what Tyson presented earlier. I think my mouse is acting up here and I’m just pulling these ten ideas from editorial in the Milbank Quarterly this year on ten ways to better understand how shifting state policy contexts affect Americans health.
I’m not going to go through all ten, but I’m going to talk about some that keep me up at night. One of the things that we have got to do a better job with is giving more attention to policy bundles or indices. There is a very strong correlation across policies within any given state. If you were to tell me a state’s minimum wage, I can tell you almost everything else about that state. I can tell you can give you a good idea of what its tobacco taxes are, or whether it has an earned income tax credit, whether they’re right to work laws. Policies are becoming increasingly bundled. And so, the tendency in a lot of our work is to create summary indices or other kinds of indices, which I think is the right direction to go. But at the same time, I think we need some general guidance on how to use these indices responsibly. And there was a really great commentary by Harper and Andy last year around their concerns of using these kinds of indices. I don’t want to persuade anyone not to use indices, particularly because there is no single policy that can explain the difference between states. We wouldn’t choose a single policy to try to describe why U.S. life expectancy is different than that in Sweden, for example, because they’re just two completely different policy context and the same is true across states.
The other thing that I want to draw attention to, and this is going to echo what Tyson said, is that going forward, we need to pay more attention to lag times. We need to understand how these state level exposures accumulate across the life course. You know, by the time people are, you know, in the 30s, 40s and 50s, they have likely experience multiple different state policy context. They might have moved and so they experienced different context, or they might have stayed in their own state of residence. But, you know, as you saw earlier, states policy contexts are very dynamic and they’re changing.
So how do we capture this cumulative exposure to different contexts over people’s life course? And at the same time, how do we understand how the health effects of those exposures also evolve over time? So, I think, you know, version 2.0 of this work is has got to get just into this messy detail about how these dynamics, how to capture the dynamic interplay across the life course. And then the last point I want to draw on here is that I think, you know, as a group of researchers interested in this topic.
We need to develop an easy to implement method for accounting for inter-state migration. It is the question that comes up any time that those of us who are doing this work present it, and there’s no agreed upon way to account for it, it’s very, very difficult to account for it. In most surveys. You just don’t have the information. But can we not come up with some sort of agreed upon way, you know, baseline way to take this into account so that we can better isolate the effects of state policies on people’s health.
That is all I have. I’m going to stop here and say thank you so much. And I look forward to your questions.
Mark Mather, PRB: Thank you so much, Jennifer. We are now going to move to the Q&A. And so, as I mentioned before, if you have a question, we have a Q&A box. You’re welcome to put your questions into the box at the bottom of the screen.
And I will start with you, Dr. Tyson. There’s a question that came in through the chat asking if you could say a bit more about why different types of structural injustice vary in their geographic distribution. This person would have expected them to align more than they do.
Dr. Tyson Brown, Duke University: Yeah, that’s a great question. So, I’ll start by just noting that, you know, in my research, I argue that because racism is dynamic, flexible and really adaptive to socio historical context, that there are likely distinct contemporary racialized regimes, and that these regimes are characterized by different manifestations and modalities of racism across place. And so, whereas contemporary discrimination, discriminatory, legal and cultural forms of racism are especially pronounced in states in Southern and Appalachian regions. Findings from my research really illustrates how contemporary structural racism that’s manifest in discriminatory institutional contexts and racial inequities, that this is particularly severe in states and Midwestern and Northeastern regions.
And so, although the historical and modern roots of place-based differences in social races have not fully understood, you know, scholars have posited that that really the extreme degrees of contemporary structural racism reflected by racial inequality and institutional contexts in many northern states, them in part from institutionalized policies and practices of social control, racialized social control through exclusion and subordination. So, you can think about examples of resource hoarding, of redlining, of racial covenants and discriminatory policing and that these white supremacist tactics were increasingly deployed after the Great Migration because northern whites perceived the increasing Black population as a threat. Right, and so, given the fluid, shapeshifting nature of structural racism, it’s really important that future research investigate the etiology and consequences of these distinct contemporary racialized regimes across time and place. But that’s a really astute observation and frankly, we don’t know and so this is a really exciting time to be doing this research and I think it’s a really important time as well.
Mark Mather, PRB: For you, Jennifer, there’s a question in the chat, to what extent do changes in interstate migration due to increasing political polarization?
I think they’re talking about people moving because of the policy context. Perhaps, would that lead to a select group of unhealthier people in more conservative states and conversely, healthier people in in liberal states? Thinking about the change in interstate migration over time.
Jennifer Karas Montez, Syracuse University: That is a great question. So, I will say that in the ways that we have tried to account for interstate migration and also the study that I mentioned in the talk, we’ve not found that it is a major contributor. So that being said, I am not sure that that will those kind of null findings will hold up post 2020 due to both increasing polarization and people potentially moving to places that are more aligned with their political values, and the fact that, you know, we’re seeing a lot of people moving to red states, to Texas, to Florida, I mean, even Oklahoma. So, I don’t know, you know, on the whole how that’s all going to play out. But my working hypothesis at this time is that what we know about who moves and who doesn’t move might be different pre versus post 2020.
Mark Mather, PRB: Thank you. This question is I guess mainly for you, Tyson. But Jennifer, you’re welcome to respond in your future directions. You mentioned digital trace data, Tyson, could you expand a little bit more on what that type of data looks like, why it may be a future direction?
Dr. Tyson Brown, Duke University: I’d be glad to. So just a level set. Digital trace data includes things like social media activity, web browsing histories, search engine queries, e-commerce transactions, text messages, emails all where we live our lives today, right? And digitally and so there’s a lot of data out there and in my work, I argue that harnessing the data revolution, which is the rapid, you know, growth in data generation and storage analysis that’s driven by technological advances big data and digitization and transforming how information is used in decision making you know in research and everyday life. Right.
So, I think this is a really important and, and it’s especially critical, to use digital trace data to really capture what we termed as backstage cultural dimensions of racism. Right. And so, you don’t have to rely upon what people responses that they give in surveys because there’s social desirability. There’s a number of reasons that we may be getting not we may not be getting the full picture from survey data, which is still really important and even administrative, that can be really important. But there’s something unique about how we live our lives online that you know, they’re basically receipts. You know your digital life doesn’t lie, right? and we have a lot of digital traces, and so I see it as really, a really valuable way to complement more traditional data sources that many of us as demographers have used.
Mark Mather, PRB: In place and again, for you at this person, notice that there’s not a lot of overlap in the map to measures of structural racism with high values in the Midwest and West, and cultural racism with high values in the southeast and on the state level similar, I guess, to the other question, but how do you envision these measures potentially capturing different pathways for racism to impact population health?
Dr. Tyson Brown, Duke University: Yeah. Well, I guess see my previous response. You know, again, getting back to these contemporary racializing regimes, I would refer to the person who asked the question to the recent study. So, there’s a 2024 article in the Journal of Health and Social Paper. Between this author by myself and Patricia Home and also a couple forthcoming in your articles and your view of sociology and review of public health will be really digging deep with some of these issues and so I think this is really important. You know, the latter part of the question was, you know, what do I see as there, you know, sort of unique or joint pathways in shaping health. Right? So, whether you have sort of the institutional manifestations and you’ve got the more ideological, cultural dimensions and that is an empirical question that we really don’t know, but many of us are working on that. And so, you know, I hope that in a year we’ll have better answer and especially five years from now. But I think that really is one of the frontiers for understanding population health in general and more broadly, and then more specifically thinking about racial inequities in health including at the state level.
Mark Mather, PRB: Your question for either or both of you, is there an agreed upon way to incorporate different races living under alternative government structure like Native Americans or tribal lands, into measures of structural racism and population health when they might not be present in our standard data resources.
Dr. Tyson Brown, Duke University: I didn’t fully understand the question, but I will just note that I think it began by is there an agreement? and I would say no. You know, these are debate, you know, like any fields of science, there are contested debates. There are new revelations, there’s discoveries, we’re always innovating and I think that we’re always learning more about these sorts of racialized practices and processes and certainly I would note that, you know, really there is no one size fits all and that there are real limitations to all the ways in which we measure these things and how folks are coded, whether it’s self-reported, whether it’s by the interviewer, whether it’s, you know, there are other ways of getting at sort of trying to triangulate to figure out someone’s racial identity or status. But, none of them are perfect. And so, this is part of the job of trying to advance the field and do so in responsible manner. So, I appreciate the question.
Mark Mather, PRB: Do you want to say anything to that, Jennifer?
Jennifer Karas Montez, Syracuse University: Sure. I’ll just add that, I mean what the question reminds me of is just, you know, the need to examine heterogeneity more carefully and, you know, the need to go below the state level to understand some of these processes. You know, there the states are just super interesting unit of analysis. I mean, you know, we call them institutional actors. And, you know, I mean, Tyson made a great pitch for why states are important, but they’re not the only thing that matters. And so, I think to get at the kinds of detailed analyses that that question would require going below the state level.
I would also say that what it seems like is that, you know, states and probably also local environments too, seem to matter most for the health of people who are marginalized. We have this term colleges of firewall, right? So that if you have a college degree or higher, it almost doesn’t matter where you live, you’re going to have good health. But with each, you know, lower level of education, that the place that you live matters more and more for shaping your health. And so, but again I mean that those kinds of analyses are going to benefit from going below the state level.
Mark Mather, PRB: Great. And I just had one question for you, Tyson. You had mentioned things like hate speech and racial animus. And I am curious about how do we measure those types of structures, partly because they might be reported differently in different local jurisdictions or not reported at all in certain places. So, I’m wondering, is that where the social media comes in to capture some of those things, or is it a combination of social media and some of the criminal justice data?
Dr. Tyson Brown, Duke University: Yeah. Great question. I think that it’s like you mentioned, you know, I think it is really important to triangulate and use a variety of data sources. They all have their strengths and limitations and certainly reporting on official Bureau of Justice statistics on hate crimes as well. It’s got its limitations, to say the least. And there’s certainly variation across place about how and when they report if they report and so that data you know, it’s got major limitations but I think that we can get at things by looking at the proportion of hate groups and indexing it to the population size. Right?
So, I think that’s really revealing, you know, when we map that data, I think that, as you mentioned also that using things like social media data really can get at some of these sorts of things by whether it’s Google searches or people’s activity on other social media platforms and there’s also experiments that folks have done, including things like the AIT that get at sort of underlying logics and subconscious biases as well as survey data. I think that, you know, there survey data on racial resentment. If you map it, it’s in a rather predictable way, it varies across states in a predictable way and it’s highly correlated with other measures of racial essentialism and norms and things along those lines. So, I think that all of the above, frankly, you know, they all have their relative advantages and disadvantages.
Mark Mather, PRB: Well, we are at time, so I think we need to close here. But Tyson and Jennifer, thank you so much for joining this discussion. It’s an important and timely topic and for those who stuck around, thanks for joining. As I mentioned, this is being recorded. We will send you a link to that recording in the next few days. So, thanks everyone.